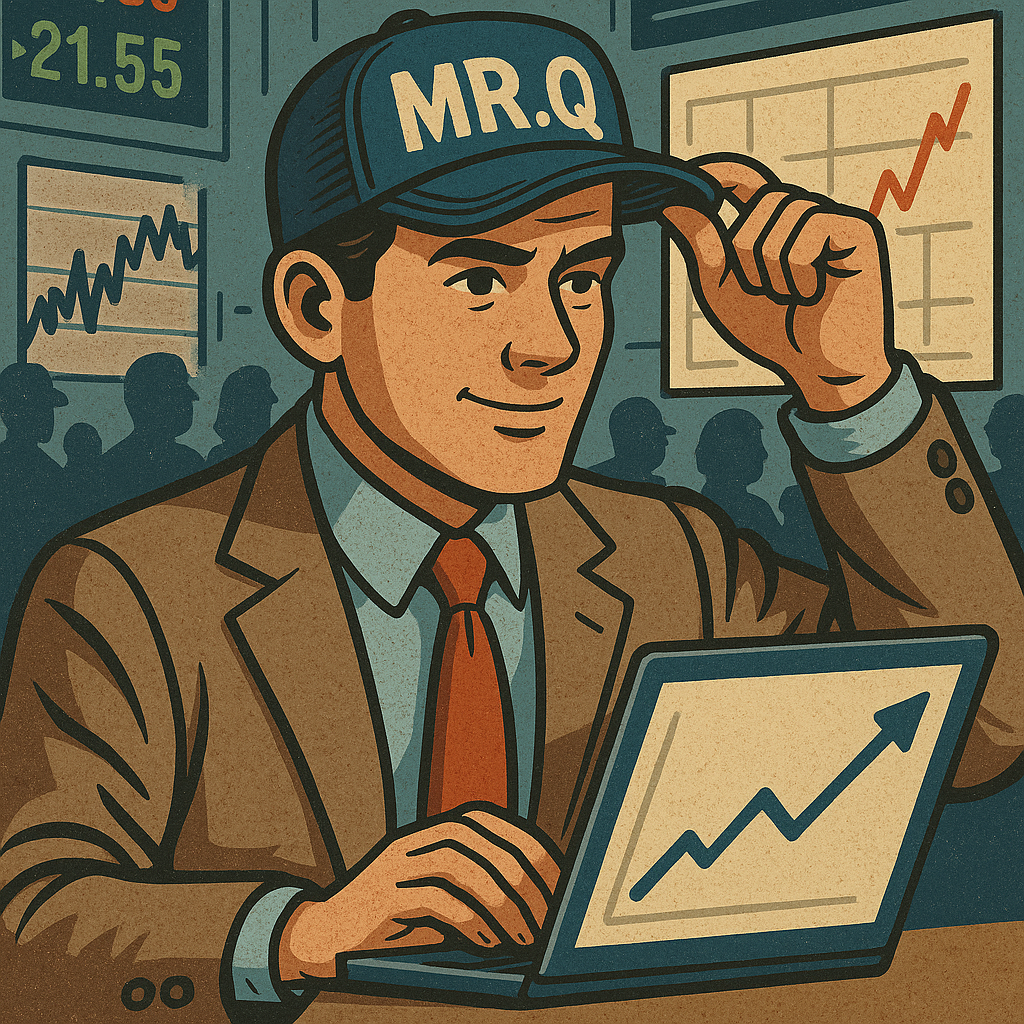
MR.Q: A New Approach to Reinforcement Learning in Finance
✨ Introduction: Real-Time Self-Tuning with MR.Q
Most machine learning models need hundreds of examples, large GPUs, and hours of training to learn anything useful. But what if you could build a system that gets smarter with just a handful of preference examples, runs entirely on your CPU, and improves while you work?
That’s exactly what MR.Q offers.
🔍 It doesn’t require full retraining.
⚙️ It doesn’t modify the base model.
🧠 It simply learns how to judge quality — and does it fast.